The issue at a high level
Artificial intelligence (AI) is becoming increasingly important for society and the economy. However, modern AI research requires expensive resources that are often only available to a small group of for-profit firms. Consequently, there is a growing concern that only a handful of companies will be able to perform cutting-edge research, leading to a worrisome concentration of power in AI development and its future.
AI has enormous potential: to make possible new discoveries in medicine and other fields; to reduce the cost of goods and services (for example, machine translation); and to improve lives. But in AI, unlike in most technologies, basic and applied research overlap—the so-called “Pasteur’s Quadrant” (Stokes 2011). An example is the Transformer architecture (Vaswani et al. 2017), an important deep-learning innovation developed by Google Brain researchers in 2017 and a crucial piece of ChatGPT. But while this invention was the product of basic research, it was almost immediately incorporated into industry models. Because of this close relationship between basic and applied research in AI, we are unlikely to see a division of labor emerge between academia and industry in AI where academia does basic research and industry focuses on commercialization. Instead, industry’s dominance will likely continue to give it an outsize impact in both basic and applied AI research going forward. Therefore, for policymakers, the key will be to ensure that academics continue to be able to participate in cutting-edge research.
Academic institutions often prioritize public interest and advancement of knowledge in their AI research and development, as opposed to solely focusing on profit maximization. This ethos can lead to AI models and research that are more attuned to societal needs and ethical considerations. However, the challenge for academic participation arises because modern AI is a resource-intensive field that relies on large datasets, talented AI scientists, and enormous computing power. Our analysis shows that these resources are concentrated in a few large, private companies. In contrast, public organizations and academic institutions lack the same resources, resulting in a lack of high-quality, public-interest models. Enabling academic researchers to play a larger, publicly-minded role in AI will require a variety of initiatives. To ensure academia has sufficient talent, academic researchers will need direct support to keep them from leaving for industry, and more open immigration policies will be needed to attract and retain promising researchers from other countries. To let academic researchers work on cutting-edge projects, investments will need to be made in public computing platforms and data. In addition to these general interventions, it will also be important to provide the resources and access for academics or other publicly minded experts to audit the most consequential industry systems.
Introduction and literature review
ChatGPT brought AI into the mainstream in a way that surprised most observers. This easy-to-use tool for generating text has the potential to transform education, healthcare, retail, and a host of other industries, but it also has its limits. It can make things up (known as “hallucinations”) and can reflect societal biases against minorities and women (Zhao et al. 2023). Additionally, the underlying training data and processes are closely held by OpenAI, which developed the tool, and Microsoft, which partially financed it, and are unavailable to the general public. Similar tools, such as Google’s Bard, also have their details kept under the veil of secrecy. Because of these restrictions, it is largely impossible to examine the decisions made in developing and deploying the model. While there are a few high-quality models that have been made publicly available, most open source models are far behind in quality.1 These facts raise concerns that the power in AI research is concentrated in the hands of the few private companies that hold enormous resources.
The societal implications of recent AI models are so great because it is considered a general-purpose technology (GPT) whose broad applicability and ability to generate complementary innovations gives it the potential to redefine existing industries and create new ones (Cockburn, Henderson, and Stern 2018). AI is also expected to play an important role in technical advances and socio-economic development (Cockburn, Henderson, and Stern 2018; Besiroglu, Emery-Xu, and Thompson 2022; Furman and Seamans 2019). For example, AI can facilitate international trade by reducing the cost of translation (Brynjolfsson et al. 2019). In the same vein, AI can cut down on medical costs by offering more affordable diagnostic tools, thus increasing accessibility (Khanna et al. 2022).
While the upsides of AI are substantial, so are the potential downsides. For example, there is a concern that industry could steer the research directions in ways that might exacerbate inequality. In particular, it might be quite economically attractive to automate tasks currently being done by humans, even though society might be better served by focusing on tasks that complement humans (Brynjolfsson 2022; Acemoglu 2021). Another concern arises from the enormous resources needed for cutting-edge AI research (Hartmann and Henkel 2020; Ahmed and Wahed 2020). These costs can create barriers to entry for start-ups and poorly resourced universities, stopping them from innovating. The resultant concentration of cutting-edge models into a few hands could also carry with it the usual ills of monopoly and monopsony: lack of competition, extraction of additional value from consumers, and even a lack of competition in R&D itself. Consequently, the National Security Commission on AI (NSCAI) concluded “[t]he consolidation of the AI industry threatens U.S. technological competitiveness” (Schmidt et al. 2021, 186).
Data and results
For decades, a typical division of labor existed between industry and academia in AI research. But more recently a small number of private companies have begun to erase this division and dominate the field (Ahmed, Wahed, and Thompson 2023) due to their greater access to data (Hartmann and Henkel 2020), computing power (Ahmed and Wahed 2020; Thompson et al. 2020), and human capital (Musser et al. 2023), giving these firms a competitive advantage in AI research. In what follows, we outline the scale of these disparities in inputs and the consequential changes in key AI outputs.
Inputs to AI research
Modern AI research depends on three key inputs:
- Data (Shokri and Shmatikov 2015). Large AI models require vast amounts of data, which can range into the billions or trillions of tokens. In deep learning, the larger the dataset used to train a model, the better it performs; more specifically, as the size of the model grows, the number of data points should grow with it (Thompson et al. 2020).
- Human capital. Talented scientists who can conduct cutting-edge research are another important ingredient. Research into deep learning-based models requires extensive experimentation, where much of the knowhow is still not codified. This tacit knowledge resides in scientists, whose skillset become particularly valuable when the demand for these professionals exceeds the available supply (Ahmed 2022).
- Computing power. Significant computing power is needed to train large AI models (Thompson et al. 2020). Research suggests that computing power has been central to recent advances in AI and its superior performance over older methods (Thompson et al. 2020; Hestness et al. 2017).
Organizations that have these key ingredients will be able to perform better in AI research by pushing the boundaries of this field, and private industry has significant advantages in all three.
Data. The large base of users of Microsoft, Google, and other giant technology companies provide them with enormous amounts of commercially valuable, structured data that academia and smaller firms cannot match. For instance, WhatsApp users sent roughly 100 billion messages a day in 2020 (Singh 2020). Industry has both the data and the ability to collect, retain, and process them, giving them an edge in exploiting that information in the training of large AI models.
Human capital. Industry has also been aggressive in assembling the talent essential for AI research. Using data from the Computing Research Association (CRA), we document private companies’ increased efforts to recruit Ph.D. holders in artificial intelligence (Ahmed, Wahed, and Thompson 2023). In 2020, nearly 70% of AI Ph.D. holders were recruited by industry, up from only 21% in 2004 (Figure 1A). The pace of this increase is greater than in most other scientific fields and is similar to that in the applied field of engineering. For a comparison, companies in the life sciences recruited roughly between 30% and 40% of Ph.D. graduates in the same time period (Ahmed, Wahed, and Thompson 2023). Furthermore, it isn’t just newly minted Ph.D.s; private companies have also ramped up their poaching of faculty AI researchers from U.S. computer science departments. This trend has seen an eightfold increase since 2006, outpacing the overall growth of computer science research faculty (Figure 1B). With the loss of Ph.D. students and faculty to industry, academic institutions are struggling to keep talent.
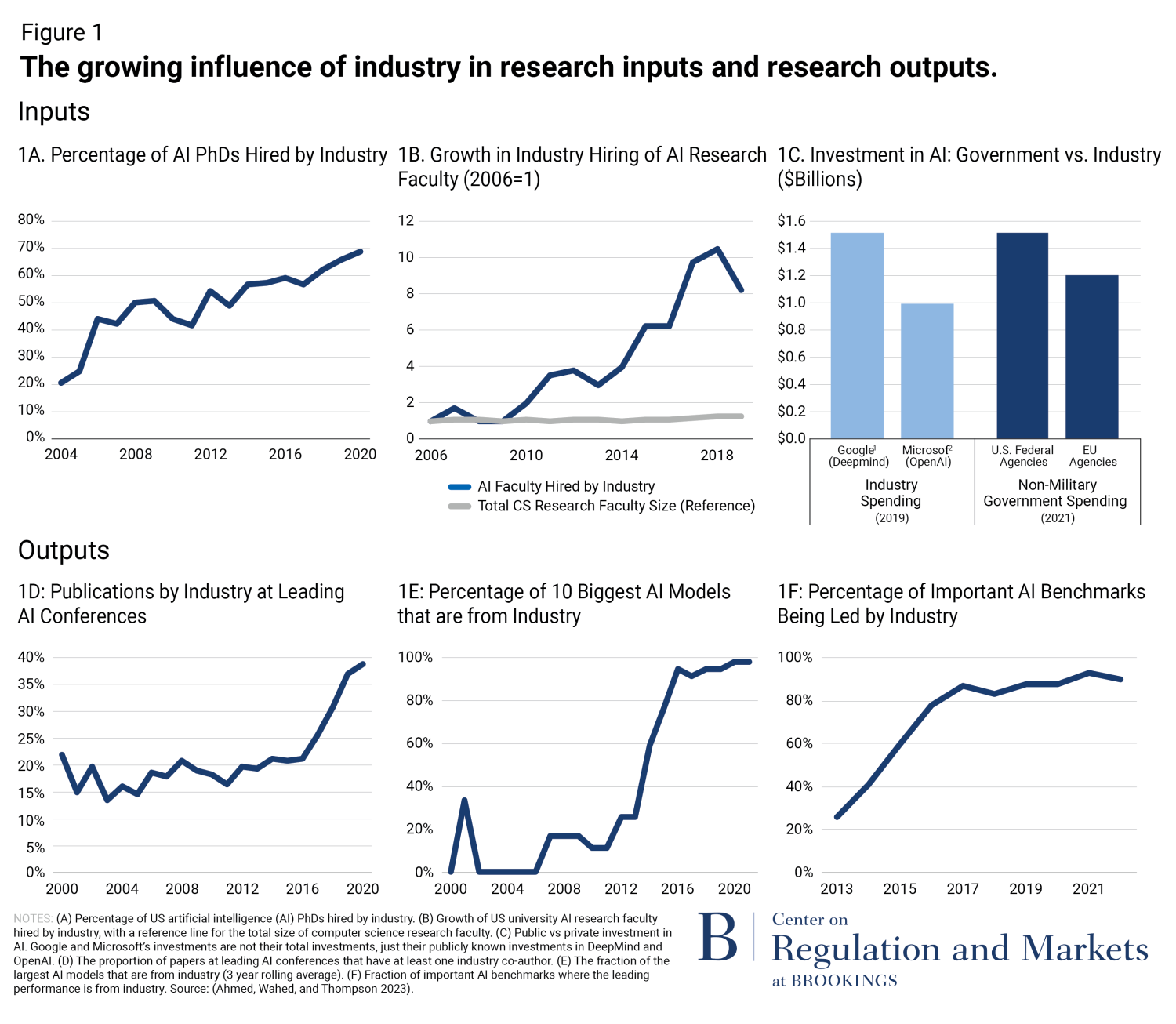
Computing power. Data also reveal the enormous disparity in computational capacity between industry and academia. Using data from ImageNet, a well-known benchmark for image recognition, we find that in 2021 industry models were on average a staggering 29 times larger than their academic counterparts (Ahmed, Wahed and Thompson 2023). Academic researchers simply do not have access to such computing power. For example, in Canada, demand from academia for the graphics processing units (GPUs) used to train machine-learning models has surged 25-fold since 2013, according to data from Canada’s National Advanced Research Computing Platform (“2022 Resource Allocations Competition Results” 2022). In recent years, the platform was only able to fill 20% of this demand.
Industry’s dominance in AI inputs is likely driven primarily by the enormous disparity in AI investments between the private and public sectors. For instance, as seen in Figure 1C, the European Union and U.S. federal agencies spent or planned to spend $1.2 billion and $1.5 billion, respectively, on AI in 2021. In contrast, Google alone spent roughly $1.5 billion in 2019 just on DeepMind, its British AI research laboratory, while Microsoft invested $1 billion in OpenAI.
Increasing dominance of industry in AI research
Industry’s supremacy in these key AI inputs has contributed to its success in AI research outcomes: publishing in leading conferences, creating the largest AI models, and dominating key benchmarks. Using a dataset of the field’s top 10 conferences, we find that in 2020 38% of the submitted papers had co-authors from industry, compared with only 22% in 2000 (Figure 1D). In the same vein, industry accounted for 96% of the biggest AI models in 2021, compared with 11% in 2010 (Figure 1E); model size is a useful proxy for the capabilities of large AI models (Thompson et al. 2020).
We also find that industry in recent years has dominated important AI benchmarks such as image recognition, sentiment analysis, language modeling, semantic segmentation, object detection, machine translation, robotics, and common-sense reasoning. As seen in Figure 1F, when we examine 20 benchmarks we find that in 2013 industry—either independently or in partnership with universities—had the leading model 26% of the time. By 2022, that had surged to industry having the leading models 90% of the time.
Policy implications
Concerns
AI is a technology that has the potential to transform numerous industries, and at the moment it is primarily being shaped by industry. This fact raises several important concerns. Consider bias: In 2018, Joy Buolamwini, an academic, and Timnit Gebru, a Microsoft employee at the time, demonstrated how commercial facial-recognition systems showed gender and racial bias in their results (Buolamwini and Gebru 2018). Auditing or external monitoring of industry by academics or nonprofit organizations, such as those in European Liability Rules for AI, might help alleviate such harms (“Liability Rules for Artificial Intelligence” 2022). However, if academics lack access to industry AI systems or the resources to create their own competing models, their capacity to decipher how industry models produce biased results or to suggest alternatives that serve public interest will be curtailed.
Industry’s dominance also threatens continued innovation and the future direction of AI research. Studies indicate that the poaching of AI faculties hurts local startup formation (Gofman and Jin 2022) and that performance on key AI benchmarks can influence the trajectory of future research (Dehghani et al. 2021). As a result, companies in this area are increasingly able to set the direction of the field.
Finally, there is the danger that the influence of industry in guiding AI research will lead to the neglect of important work aimed at serving the public good. Recent work suggests that private-sector AI professionals typically focus on deep-learning techniques that require large amounts of data and extensive computational power and that they do this at the cost of considering the societal and ethical implications of AI and developing applications for sectors such as health care (Klinger, Mateos-garcia, and Stathoulopoulos 2020). Accordingly, a concern is that industry researchers will be less likely to reflect society’s priorities since profit maximization via the commercialization of science is a key priority for firms. Therefore, we might observe more AI-induced inequality and job displacement in the near future. Indeed, some scholars (Brynjolfsson 2022; Acemoglu 2021) worry that the current trajectory of research prioritizes replacing human work with AI over using it to enhance human abilities.
Suggested policy solutions
These trends, we believe, can be altered by equipping academics with the necessary resources to create AI models in the public interest.
Access to computing. One way to do this is by providing cloud-computing resources to academics and startup enterprises. Fortunately, efforts are underway in the United States to establish the National AI Research Resource (NAIRR), which would provide AI researchers and students with access to computational resources, high-quality datasets, and other tools (Ho et al. 2021). However, the financial commitment to this initiative is $2.6 billion over a six-year period (Coldewey 2023), which remains modest in comparison to overall spending by industry.
Supporting academics. Enabling top researchers to stay in academia could also help universities to compete with industry. The Canada Research Chair program, for example, provides additional funds to pay competitive faculty salaries and grants to select researchers, enabling the country to recruit and retain top academics (“Canada Research Chairs” 2023). A similar initiative in the U.S. could help deter industry poaching of AI research faculty.
Immigration. Talent is a crucial asset in AI research (Musser et al. 2023), and its scarcity is a significant concern (Ahmed 2022; Gofman and Jin 2022). One strategy to address this issue could be for the U.S. to welcome more immigrants with relevant expertise. This approach could potentially broaden the pool of talent sufficiently that organizations that cannot currently compete with large industry players could still recruit high-quality AI researchers.
Increasing diversity. Lack of diversity among those developing AI is an important issue (West, Whittaker, and Crawford 2019), and greater effort is needed to diversify the AI workforce. A more representative workforce, research suggests, is more likely to pursue often-overlooked subjects (Einiö, Feng and Jaravel 2019); one study found that women scientists produced more biomedical patents that were focused on women’s health (Koning, Samila, and Ferguson 2021). A more diverse workforce in AI could facilitate innovation in areas that are currently underserved (e.g., machine translations that can serve underserved communities). More international cooperation across countries, especially with Global South countries could also help unlock hidden talent and increase diversity in the workforce.
Conclusion
The role of AI in society has grown by leaps and bounds in the past decade. As private resources have poured into this area, academics have lost ground and consequently have lost their ability to be a publicly-minded counterweight. While such a counterweight isn’t needed when industry’s goals align with society’s, this isn’t always true. To ensure that academia can play a public-minded role when this is not the case, we will need policy interventions to ensure that academia has all the key ingredients of AI research: large datasets, skilled scientists, and significant computing power. Absent such interventions, AI research and the influential AI models that flow from them will reside mostly in the hands of a few private companies.
References
“2022 Resource Allocations Competition Results.” 2022. Digital Research Alliance of Canada. https://alliancecan.ca/en/services/advanced-research-computing/accessing-resources/resource-allocation-competitions/2022-resource-allocations-competition-results.
Acemoglu, Daron. 2021. “Harms of AI.” https://economics.mit.edu/sites/default/files/publications/Harms%20of%20AI.pdf.
Ahmed, Nur. 2022. “Competitive Scientific Labor Market and Firm-Level Appropriation Strategy in Artificial Intelligence Research.” MIT Sloan Working Paper. https://mackinstitute.wharton.upenn.edu/wp-content/uploads/2023/11/Ahmed-Nur_Scientific-Labor-Market-and-Firm-level-Appropriation-Strategy-in-Artificial-Intelligence-Research.pdf
Ahmed, Nur, and Muntasir Wahed. 2020. “The De-Democratization of AI: Deep Learning and the Compute Divide in Artificial Intelligence Research.” arXiv. http://arxiv.org/abs/2010.15581.
Ahmed, Nur, Muntasir Wahed, and Neil C Thompson. 2023. “The Growing Influence of Industry in AI Research.” Science 379 (6635). American Association for the Advancement of Science: 884–86. https://doi.org/10.1126/science.ade2420.
Besiroglu, Tamay, Nicholas Emery-Xu, and Neil Thompson. 2022. “Economic Impacts of AI-Augmented R&D.” arXiv. http://arxiv.org/abs/2212.08198.
Brynjolfsson, Erik. 2022. “The Turing Trap: The Promise & Peril of Human-Like Artificial Intelligence.” Daedalus 151 (2): 272–87. https://doi.org/10.1162/daed_a_01915.
Buolamwini, Joy, and Timnit Gebru. 2018. “Gender Shades: Intersectional Accuracy Disparities in Commercial Gender Classification.” In Proceedings of the 1st Conference on Fairness, Accountability and Transparency, edited by Sorelle A. Friedler and Christo Wilson, 81:77–91. Proceedings of Machine Learning Research. PMLR. https://proceedings.mlr.press/v81/buolamwini18a.html.
“Canada Research Chairs.” 2023. Government of Canada. November 14, 2023. https://www.chairs-chaires.gc.ca/home-accueil-eng.aspx.
Cockburn, Iain M, Rebecca Henderson, and Scott Stern. 2018. “The Impact of Artificial Intelligence on Innovation.” NBER Working Paper. https://www.nber.org/papers/w24449.
Coldewey, Devin. 2023. “Task Force Proposes New Federal AI Research Outfit with $2.6B in Funding.” TechCrunch. January 24, 2023. https://techcrunch.com/2023/01/24/task-force-proposes-new-federal-ai-research-outfit-with-2-6b-in-funding/.
Furman, Jason, and Robert Seamans. 2019. “AI and the Economy.” Innovation Policy and the Economy 19 (January): 161–91. https://doi.org/10.1086/699936.
Gofman, Michael, and Zhao Jin. 2022. “Artificial Intelligence, Human Capital, and Innovation.” SSRN Electronic Journal. https://doi.org/10.2139/ssrn.3449440.
Hartmann, Philipp, and Joachim Henkel. 2020. “The Rise of Corporate Science in AI: Data as a Strategic Resource.” Academy of Management Discoveries 6 (3). https://doi.org/10.5465/amd.2019.0043.
Hestness, Joel, Sharan Narang, Newsha Ardalani, Gregory Diamos, Heewoo Jun, Hassan Kianinejad, Md Patwary, Mostofa Ali, Yang Yang, and Yanqi Zhou. 2017. “Deep Learning Scaling Is Predictable, Empirically.” arXiv. http://arxiv.org/abs/1712.00409.
Ho, Daniel E., Jennifer King, Russell C. Wald, and Christopher Wan. 2021. “Building a National AI Research Resource: A Blueprint for the National Research Cloud.” Stanford Institute for Human-Centered Artificial Intelligence. https://hai.stanford.edu/white-paper-building-national-ai-research-resource.
Khanna, Narendra N., Mahesh A. Maindarkar, Vijay Viswanathan, Jose Fernandes E Fernandes, Sudip Paul, Mrinalini Bhagawati, Puneet Ahluwalia, et al. 2022. “Economics of Artificial Intelligence in Healthcare: Diagnosis vs. Treatment.” Healthcare 10 (12): 2493. https://doi.org/10.3390/healthcare10122493.
Klinger, Joel, Juan Mateos-garcia, and Konstantinos Stathoulopoulos. 2020. “A Narrowing of AI Research?” arXiv. http://arxiv.org/abs/2009.10385.
Koning, Rembrand, Sampsa Samila, and John Paul Ferguson. 2021. “Who Do We Invent for? Patents by Women Focus More on Women’s Health, but Few Women Get to Invent.” Science 372 (6548): 1345–48. https://doi.org/10.1126/science.aba6990.
Musser, Micah, Rebecca Gelles, Catherine Aiken, and Andrew Lohn. 2023. “‘The Main Resource Is the Human.’” Center for Security and Emerging Technology. https://doi.org/10.51593/20210071.
Schmidt, Eric, Robert Work, Safra Catz, Eric Horvitz, Steve Chien, Andrew Jassy, Mignon Clyburn, et al. 2021. “The National Security Commission on Artificial Intelligence.” National Security Commission on Artificial Intelligence. https://www.nscai.gov/2021-final-report/.
Singh, Manish. 2020. “WhatsApp is now delivering roughly 100 billion messages a day.” TechCrunch. October 29, 2020. https://techcrunch.com/2020/10/29/whatsapp-is-now-delivering-roughly-100-billion-messages-a-day/.
Shokri, Reza, and Vitaly Shmatikov. 2015. “Privacy-Preserving Deep Learning.” In Proceedings of the 22nd ACM SIGSAC Conference on Computer and Communications Security, 1310–21. Denver Colorado USA: ACM. https://doi.org/10.1145/2810103.2813687.
Stokes, Donald E. 1997. Pasteur’s Quadrant: Basic Science and Technological Innovation. Washington, DC: Brookings Institution Press.
Thompson, Neil C., Kristjan Greenewald, Keeheon Lee, and Gabriel F. Manso. 2020. “The Computational Limits of Deep Learning.” arXiv. http://arxiv.org/abs/2007.05558.
West, Sarah Myers, Meredith Whittaker, and Kate Crawford. 2019. “Discriminating Systems: Gender, Race And Power in AI.” AI Now Institute. https://ainowinstitute.org/publication/discriminating-systems-gender-race-and-power-in-ai-2.
Vaswani, Ashish, Noam Shazeer, Niki Parmar, Jakob Uszkoreit, Llion Jones, Aidan N Gomez, Łukasz Kaiser, and Illia Polosukhin. 2017. “Attention Is All You Need.” In Advances in Neural Information Processing Systems, edited by I. Guyon, U. Von Luxburg, S. Bengio, H. Wallach, R. Fergus, S. Vishwanathan, and R. Garnett. Vol. 30. Curran Associates, Inc. https://proceedings.neurips.cc/paper_files/paper/2017/file/3f5ee243547dee91fbd053c1c4a845aa-Paper.pdf.
Zhao, Wayne Xin, Kun Zhou, Junyi Li, Tianyi Tang, Xiaolei Wang, Yupeng Hou, Yingqian Min, et al. 2023. “A Survey of Large Language Models.” arXiv. http://arxiv.org/abs/2303.18223.
-
Acknowledgements and disclosures
The FutureTech project at MIT is supported by grants from Accenture, Microsoft, IBM, Good Ventures, the MIT-AF accelerator, MIT Lincoln Laboratory and the National Science Foundation. The authors did not receive financial support from any firm or person for this article or, other than the aforementioned, from any firm or person with a financial or political interest in this article. The authors are not currently an officer, director, or board member of any organization with a financial or political interest in this article.
-
Footnotes
- The rankings provided by the Large Model System Organization show that the top six performing models ranked by aggregate scores on a number of benchmarks and by user ratings are all proprietary models. See https://chat.lmsys.org/?leaderboard
The Brookings Institution is committed to quality, independence, and impact.
We are supported by a diverse array of funders. In line with our values and policies, each Brookings publication represents the sole views of its author(s).